Understanding The Impact Of Neural Networks On Modern AI
Neural networks have revolutionized the landscape of artificial intelligence (AI), driving innovation across various sectors. This article delves into the intricate workings of neural networks, their applications, and their transformative effects on industries. With the growing reliance on AI technologies, understanding neural networks becomes essential for anyone looking to stay ahead in this fast-paced digital world.
As we explore the depths of neural networks, we will uncover their fundamental principles, the different types, and the challenges they face. By the end of this article, you will have a comprehensive understanding of how neural networks function and their significance in shaping the future of technology.
Join us as we embark on this enlightening journey through the fascinating realm of neural networks, examining their role in modern AI and the potential they hold for the future.
- Exploring The Impact Of Documentary About Piddy A Comprehensive Analysis
- Liam Payne A Stars Journey On Americas Got Talent
Table of Contents
- What Are Neural Networks?
- How Do Neural Networks Work?
- Types of Neural Networks
- Applications of Neural Networks
- Challenges and Limitations
- The Future of Neural Networks
- Conclusion
- References
What Are Neural Networks?
Neural networks are a subset of machine learning algorithms that are inspired by the structure and function of the human brain. They consist of interconnected layers of nodes, or "neurons," that process information in a manner similar to biological neurons. This architecture enables neural networks to learn from data, recognize patterns, and make predictions.
How Do Neural Networks Work?
The functioning of neural networks revolves around the concept of learning from data through a process known as training. Training involves adjusting the weights of connections between neurons based on the input data and the corresponding output. This is achieved through an algorithm called backpropagation, which minimizes the error in predictions.
Key Steps in Training Neural Networks:
- Initialization: Assigning initial weights to the connections between neurons.
- Forward Propagation: Passing input data through the network to generate output.
- Loss Calculation: Measuring the difference between the predicted output and the actual output.
- Backpropagation: Updating the weights based on the computed loss.
Types of Neural Networks
Neural networks come in various forms, each suited for specific tasks and applications. Understanding the differences between these types is crucial for effectively leveraging their capabilities.
- Kathy Bates The Queen Of American Horror Story
- All You Need To Know About Fox 59 News Your Ultimate Guide
Feedforward Neural Networks
Feedforward neural networks are the simplest type, where information moves in one direction—from input to output. They are commonly used for classification tasks.
Convolutional Neural Networks
Convolutional neural networks (CNNs) are designed for processing structured grid data, such as images. They utilize convolutional layers to automatically learn spatial hierarchies of features.
Recurrent Neural Networks
Recurrent neural networks (RNNs) are specialized for sequential data, allowing information to persist across time steps. They are widely used in natural language processing and time series prediction.
Applications of Neural Networks
Neural networks have found applications across numerous domains, demonstrating their versatility and effectiveness. Some notable applications include:
- Image Recognition: Neural networks excel in identifying and classifying images.
- Natural Language Processing: They power language translation, sentiment analysis, and chatbots.
- Healthcare: Neural networks assist in diagnosing diseases and personalizing treatment plans.
- Finance: They are used for fraud detection and algorithmic trading.
Challenges and Limitations
Despite their remarkable capabilities, neural networks face several challenges that can hinder their effectiveness:
- Overfitting: When a model learns noise in the training data rather than the underlying distribution.
- Data Requirements: Neural networks require large amounts of data for effective training.
- Computational Resources: Training complex networks demands significant computational power.
The Future of Neural Networks
The future of neural networks looks promising, with ongoing research and advancements in deep learning techniques. Innovations such as transfer learning, which allows models to leverage knowledge from one task to improve performance on another, hold great potential. Additionally, the integration of neural networks with other AI technologies will likely lead to even more sophisticated applications.
Conclusion
In conclusion, neural networks play a pivotal role in the advancement of artificial intelligence, transforming how we interact with technology. Their ability to learn from data and make predictions has opened up new avenues in various fields. As we continue to explore and develop these technologies, understanding their workings and implications becomes essential for harnessing their full potential.
We encourage you to leave your thoughts in the comments section below, share this article with others interested in AI, and explore more insightful articles on our website.
References
- Goodfellow, I., Bengio, Y., & Courville, A. (2016). Deep Learning. MIT Press.
- LeCun, Y., Bengio, Y., & Haffner, P. (1998). Gradient-Based Learning Applied to Document Recognition. Proceedings of the IEEE.
- Rumelhart, D. E., Hinton, G. E., & Williams, R. J. (1986). Learning Representations by Back-Propagating Errors. Nature.
- David Byrne Net Worth 2024 An Indepth Analysis
- The Simpsons Episode Piddy A Deep Dive Into The Iconic Animation
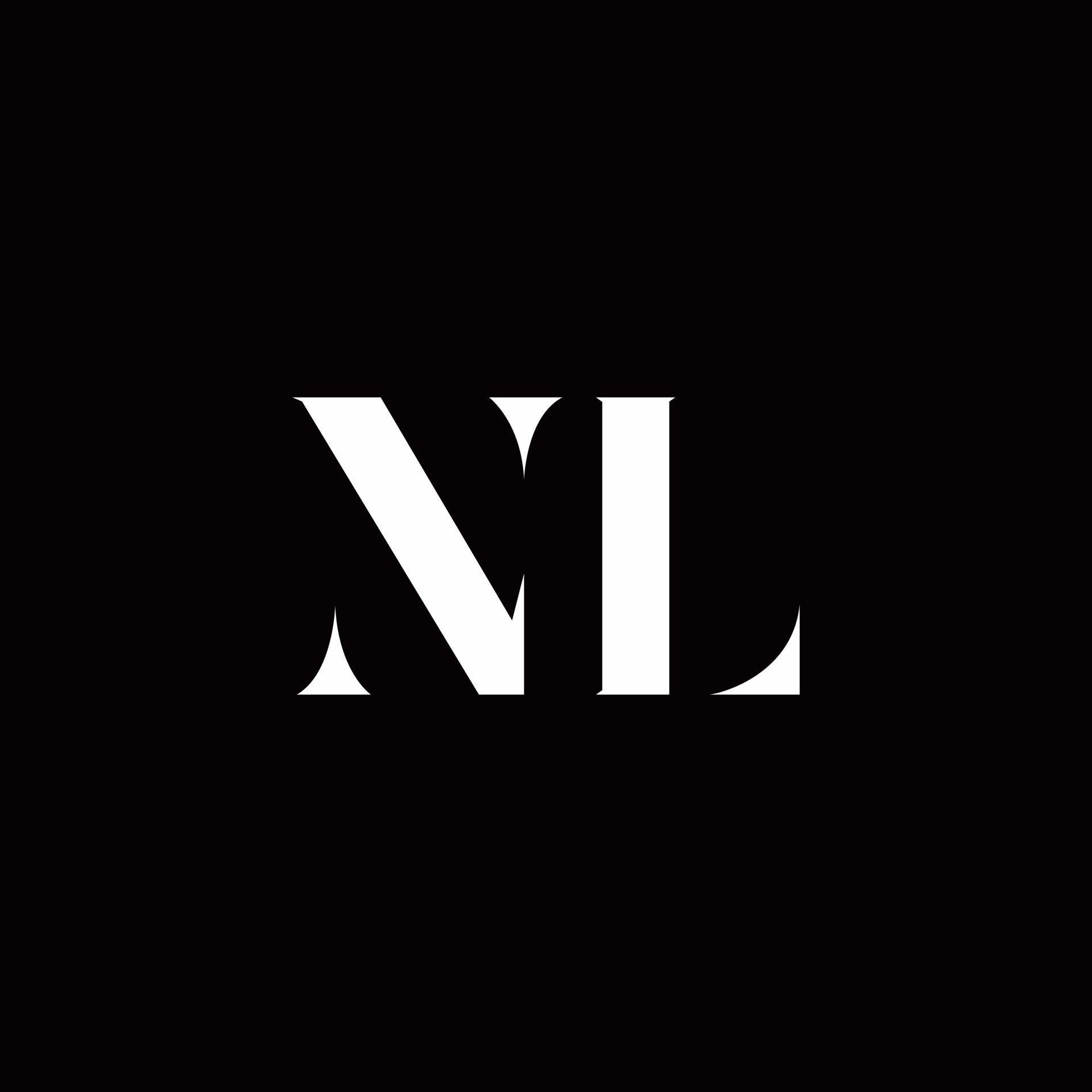
NL Logo Letter Initial Logo Designs Template 2767865 Vector Art at Vecteezy
New NL Branding Publicatie Agroberichten Buitenland
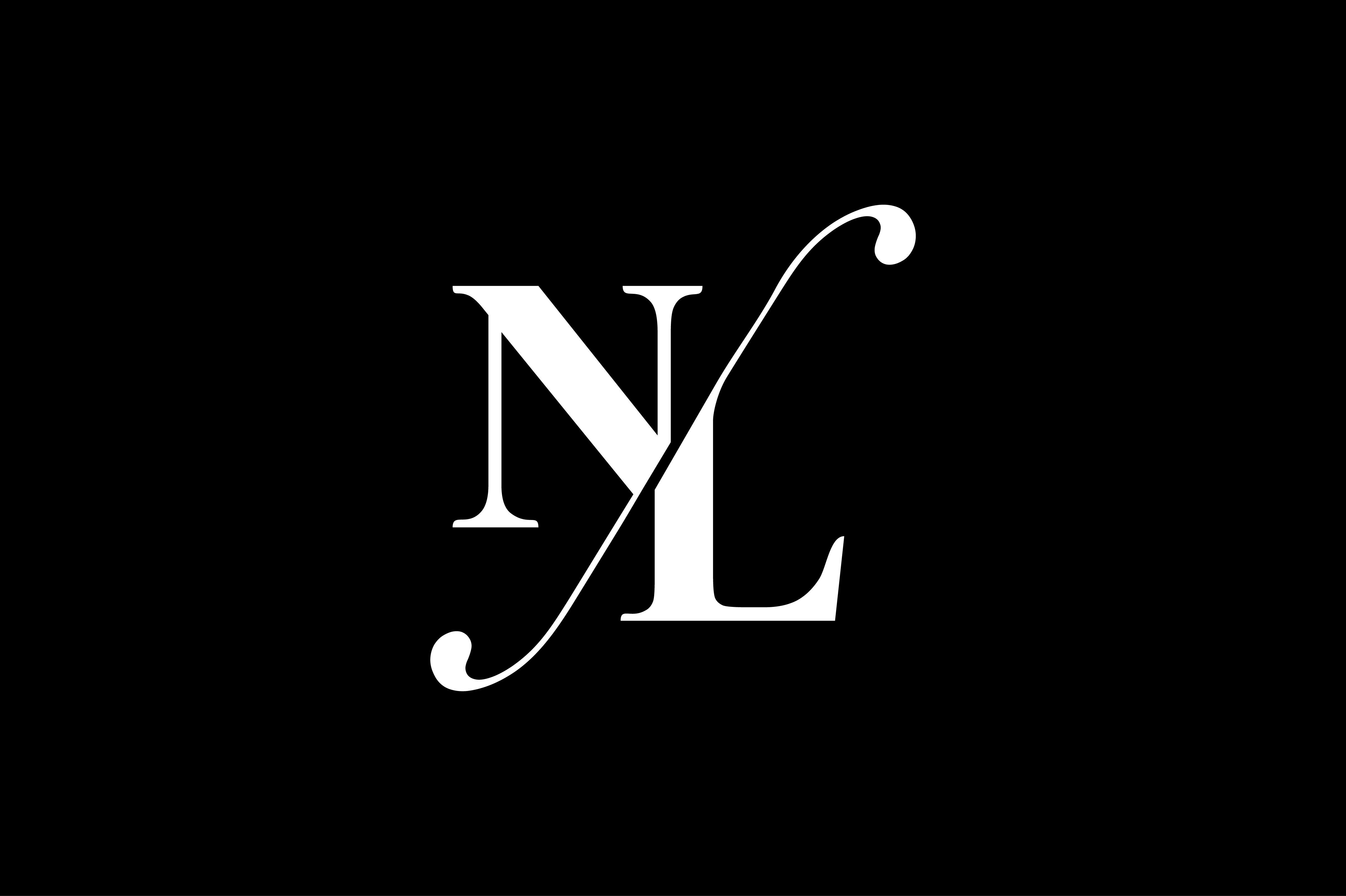
NL Monogram Logo design By Vectorseller