Understanding Point Net: The Future Of Point Cloud Processing In 3D Data Analysis
Point Net has emerged as a revolutionary architecture designed specifically for processing point clouds, which are crucial in various fields such as robotics, autonomous driving, and 3D modeling. With the increasing need for accurate 3D data analysis, Point Net serves as a game changer in how we interpret and utilize point cloud data. In this article, we will delve deep into the intricacies of Point Net, exploring its architecture, applications, and the significant impact it has on the technology landscape.
The advancement of technologies in artificial intelligence and machine learning has paved the way for innovative solutions in point cloud processing. Point Net stands out by addressing the unique challenges presented by unordered point sets, providing a robust framework for efficient data processing. This article will discuss the foundational concepts behind Point Net, its implementations, and future prospects in the industry.
As we move forward in this digital era, understanding the significance of Point Net becomes essential for professionals and enthusiasts alike. We aim to provide a comprehensive overview that not only highlights the technical aspects but also showcases real-world applications and benefits. Let’s embark on this journey to uncover the potential of Point Net in shaping the future of 3D data analysis.
- Yeon Woo Jin The Rising Star Of Korean Entertainment
- Exploring The Impact Of Documentary About Piddy A Comprehensive Analysis
Table of Contents
- What is Point Net?
- Architecture of Point Net
- Applications of Point Net
- Benefits of Using Point Net
- Challenges in Point Cloud Processing
- Future of Point Net
- Case Studies Using Point Net
- Conclusion
What is Point Net?
Point Net is a neural network architecture specifically designed to process point cloud data, which consists of sets of data points in a three-dimensional coordinate system. Unlike traditional deep learning methods that operate on grid-like data structures (such as images), Point Net can handle unordered sets of points, making it particularly effective for 3D object recognition and segmentation.
Key Features of Point Net
- Handles unordered point sets efficiently.
- Learn features directly from raw point cloud data.
- Robust to noise and outliers.
- Scalable to large point cloud datasets.
Architecture of Point Net
The architecture of Point Net is composed of several layers that work together to process point cloud data effectively. The primary components include:
1. Input Transformation
This layer normalizes the input point cloud data, ensuring that the data is centered and scaled properly for subsequent processing.
- Does Rob Zombie Have Children Discovering The Family Life Of The Rock Icon
- Exploring The Life And Journey Of Tim Duncans Wife A Deep Dive
2. Feature Extraction
Point Net employs a series of multi-layer perceptrons (MLPs) to extract features from each point independently. This allows the network to learn unique features from each point without the need for a predefined structure.
3. Global Feature Aggregation
After feature extraction, the network aggregates the features from all points to create a global feature vector. This step captures the overall information from the entire point cloud.
4. Output Transformation
The final output layer is responsible for making predictions, whether it's classification, segmentation, or other tasks. This layer utilizes the global feature vector to derive meaningful insights from the processed data.
Applications of Point Net
Point Net has a wide range of applications across various industries, showcasing its versatility and effectiveness in handling 3D data.
1. Autonomous Vehicles
In the realm of autonomous driving, Point Net is used for recognizing and interpreting objects in the vehicle's environment, enabling safer navigation and decision-making.
2. Robotics
Robots utilize Point Net for tasks such as object manipulation, navigation, and environment mapping, allowing them to interact effectively with their surroundings.
3. Augmented and Virtual Reality
Point Net plays a crucial role in creating realistic 3D models for AR and VR applications, enhancing the user experience and immersion in digital environments.
Benefits of Using Point Net
The adoption of Point Net in various applications brings several benefits:
- Improved accuracy in 3D data interpretation.
- Reduced computational requirements compared to traditional methods.
- Enhanced ability to process large and complex point cloud datasets.
- Greater robustness to noise and data irregularities.
Challenges in Point Cloud Processing
Despite its advantages, Point Net faces several challenges that need to be addressed:
- Handling large-scale point clouds can be computationally intensive.
- Effectively managing noise and outliers in real-world data.
- Optimizing the architecture for specific applications may require extensive tuning.
Future of Point Net
The future of Point Net looks promising as researchers and developers continue to explore its potential. Ongoing advancements in machine learning and neural network architectures will likely lead to enhanced versions of Point Net, offering improved performance and efficiency.
Case Studies Using Point Net
A number of case studies highlight the successful implementation of Point Net in real-world scenarios:
- An autonomous vehicle company leveraging Point Net for real-time object detection.
- A robotics research lab using Point Net for precise object manipulation in cluttered environments.
- A gaming company utilizing Point Net to create realistic 3D environments for VR experiences.
Conclusion
Point Net represents a significant advancement in the processing of point cloud data, enabling various applications across multiple industries. Its unique architecture allows for effective handling of unordered point sets, making it an essential tool for modern technology. As we continue to explore its capabilities, the potential applications of Point Net are limitless. We encourage readers to share their thoughts on Point Net and its impact on 3D data analysis in the comments below.
Thank you for reading! We hope you found this article informative and insightful. Don’t forget to check out our other articles for more in-depth discussions on similar topics.
- Sidney Crosbys Kids A Deep Dive Into His Family Life
- Piddy Beat 0 To 100 The Rise Of A Music Phenomenon
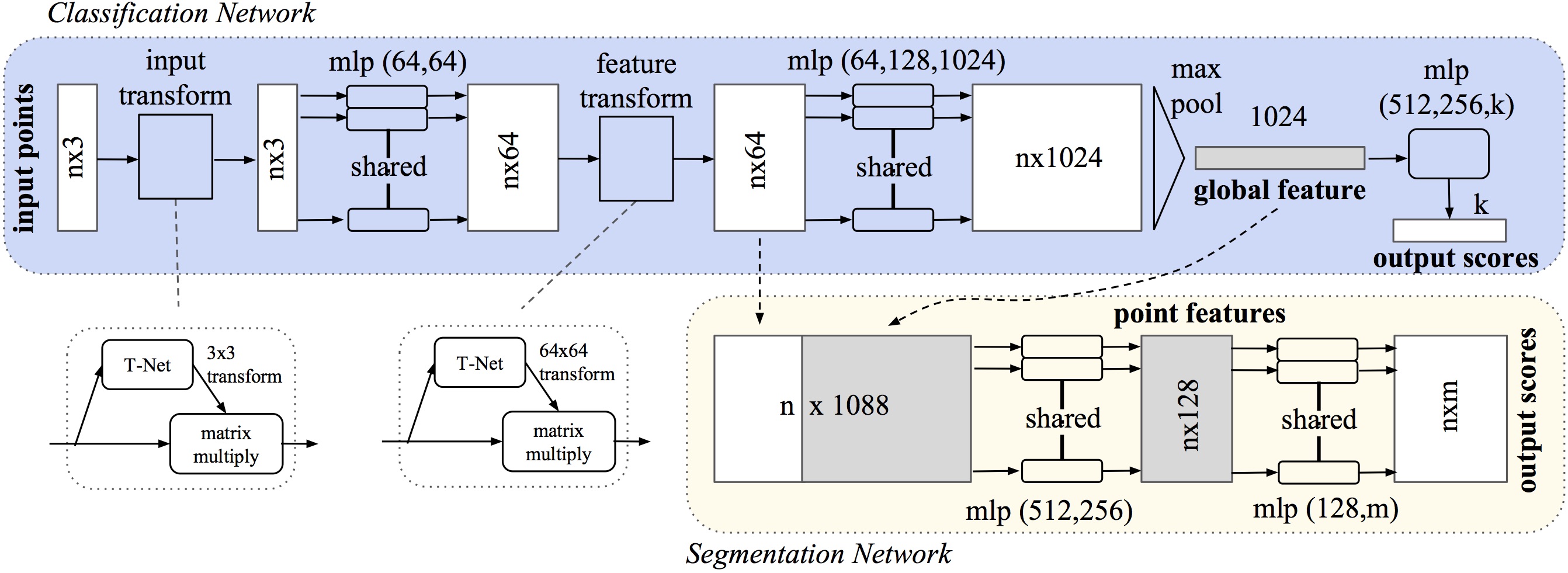
Understanding of network architecture TechNotes
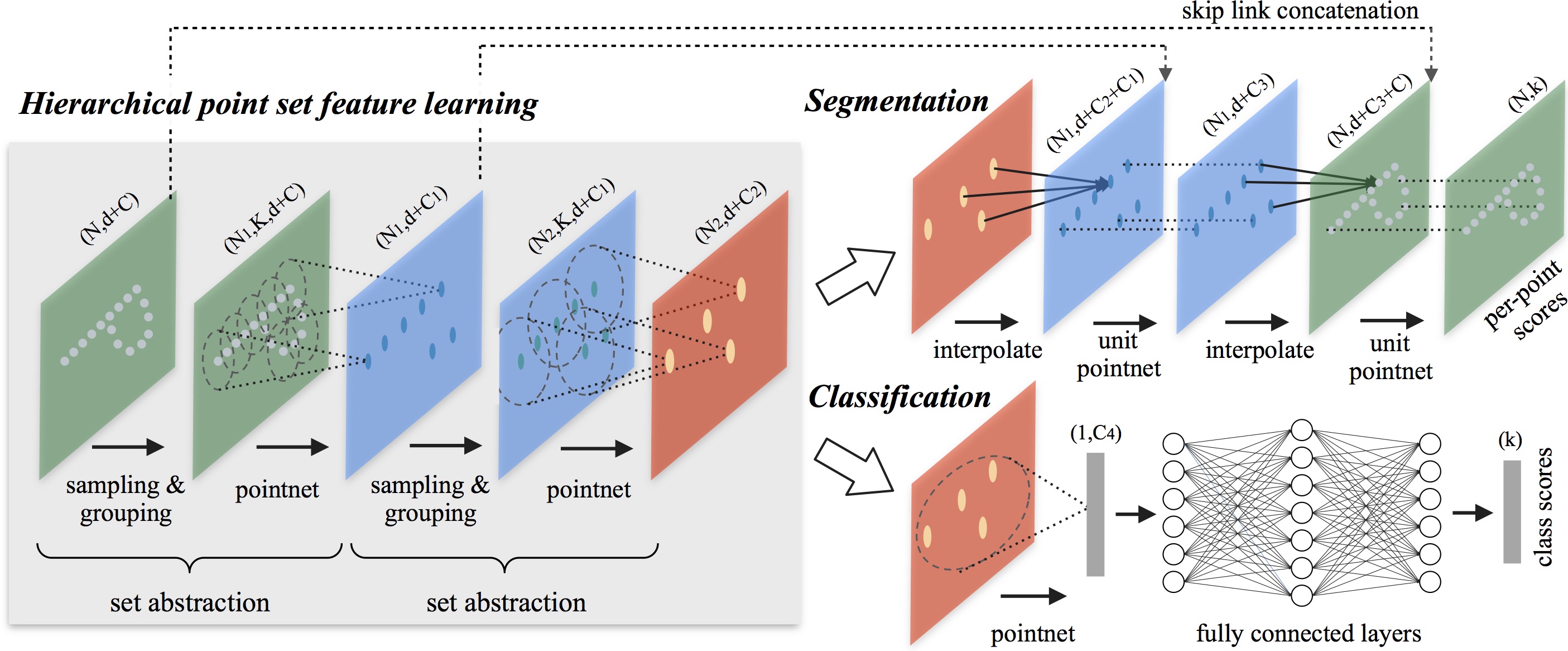
On point clouds Semantic Segmentation Open3D
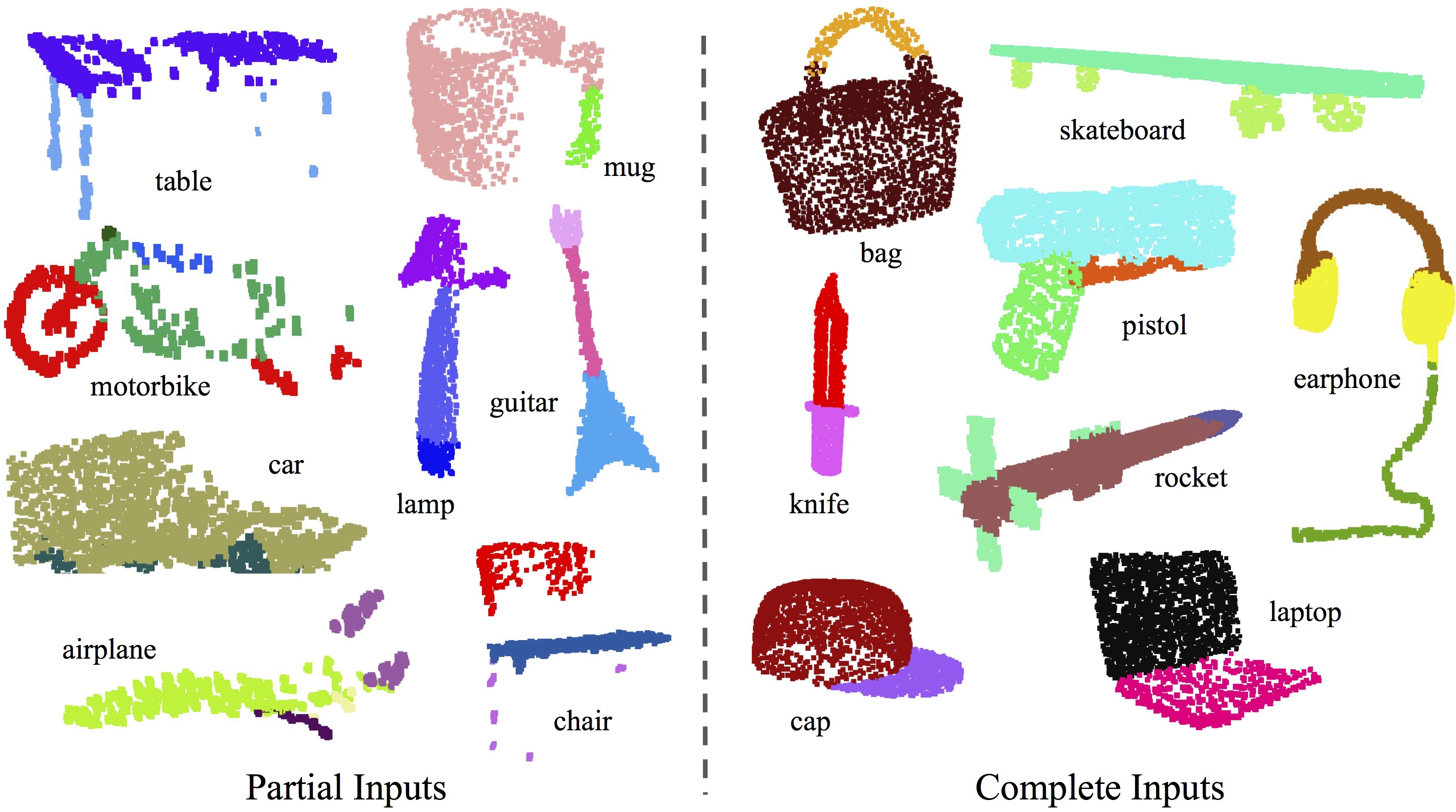